Generative AI-Powered Call Scoring: Benefits & How To Setup [Video]
When deciding on quality management solutions for your contact center, like MiaRec’s Automated Quality Management (AQM) solutions, you wonder how you can use them to get the best ROI.
At MiaRec, we customize our AQM solutions to meet contact center demands across industries, including healthcare, finance, retail, and more. We believe in catering our AQM solutions to your contact center’s specific quality assurance needs.
In this article and video, you will learn how MiaRec's new generative AI-powered Auto Call Scoring (Auto QA) will revolutionize your QA processes. I will walk you through and show you how it works, how accurate it is, and share some tips on how you can instantly improve agent performances and customer experiences.
The Challenge of Manual Call Evaluation & The Power of Generative AI Auto Call Scoring
Traditionally, contact centers have relied on manual call evaluations. This method is time-consuming and often only provides insights into a small fraction of the total call volume, typically around 1-5%. This limited visibility can hinder the ability to identify areas of improvement and provide consistent quality across all calls.
MiaRec's new generative AI-powered Auto QA offers a solution to this challenge. This technology can automatically and accurately evaluate and score 100% of calls. The generative AI model understands the context within conversations, providing relevant insights without any configuration required by the end user. This means that contact centers don't need a team of data analysts or AI engineers to benefit from this feature.
Benefits of Generative AI Auto Call Scoring
-
Precision: The generative AI evaluates calls with high accuracy, ensuring that the evaluations reflect the actual quality of the call.
-
Contextual Insights: For every question, the AI provides context, explaining why a particular answer was chosen. This eliminates the need to listen to calls or scan transcripts for context.
-
Comprehensive Coverage: Unlike manual evaluations, which cover a small percentage of calls, this technology evaluates every single call, offering a complete picture of agent performance.
-
The system requires no configuration from the end user, making it user-friendly.
-
The system provides contextually relevant answers and insights.
-
The system allows for the creation of multiple scorecards for different departments within a contact center.
-
The QA dashboard provides a comprehensive view of agent performance, enabling managers to identify top and bottom performers for targeted coaching.
-
The system provides trend analysis to track agent performance over time.
Setting Up AI-Driven Auto QA
The process begins by constructing a scorecard personalized for your company and any subset of departments, i.e., customer service, sales, etc. Here you can use questions from previous scorecards you've created or make new ones, and you can craft the questions using normal language.
Image: Screenshot of sample scorecard questions. You can customize and add your own questions to be scored by the Generative AI model.
For instance, you can ask simple questions like "Did the agent use a polite greeting?" or more complex questions like "Did the agent actively listen to the customer's concerns and answer all the customer's questions without the customer having to repeat themselves?"
Create as many sections and questions as needed for each department you are creating a scorecard for. Unlike models based on parameters such as "Said the following greetings (list of multiple acceptable greetings) in the first X number of words", there are no complicated rules to create or thresholds to be met. There is no need to employ or contract a team of data analysts or engineers to create, test, and fine-tune these rules. The generative AI model understands the context of the whole conversation and gives contextually relevant answers, which it learned from thousands of hours of previous call conversations.
How to set up questions
Now, we will outline the steps to create your scorecards, which you can also watch in the video above. First, from the MiaRec platform, navigate to the QA tab and then to the form designer section. Here is where a user can add sections and questions to the scorecard. For each question
- Type in the query
- Assign it to the AI assistant
- Type in the possible answer choices
- Assign a value to each answer
Then hit save and move on to the next question. It's simple and easy to use. After assigning the questions to the AI assistant, the generative AI then takes over, evaluating calls based on these questions without the need for those complex configurations or query expressions I mentioned earlier.
Image: Screenshot of an example question that is part of a MiaRec Generative AI Auto QA Scorecard.
After you have finished your scorecard, 100% of your calls will be evaluated by the AI, which then allows you to drill down into areas that need improvement or see why some departments excel in certain areas.
An important note is that you can create as many scorecards as you would like. For example, you can create one scorecard for your Sales Department and one scorecard for your Customer Service Department.
In addition to the flexibility of creating multiple scorecards, our AI-powered Auto QA offers you the ability to tailor the analysis even further. You can designate specific scorecards to evaluate calls based on predefined conditions. For instance, you can instruct the system to have your 'Customer Service Scorecard' specifically analyze calls made by your customer service team, ensuring that the evaluation is finely tuned to their unique responsibilities and challenges. Similarly, you can set up your 'Sales Scorecard' to focus exclusively on calls made by your sales team, allowing for a specialized assessment of their interactions.
Example of an analyzed call
After you have input all the questions into the scorecard and the generative AI starts analyzing calls, you can now gain valuable insight from the AI scoring. Let's take a deeper look.
Besides getting aggregate scores on each department, group, division, etc., you can also look at each agent and any individual call that they were a part of.
Some of the data the analyzed report gives you is the total score, which is a percentage out of 100, a list of each question with its point score, and the reason for the score. As you can see from the screenshot, the AI recognizes simple interactions like customer name, email, zip code, etc.
Image: Screenshot of a completed evaluation report analyzed by MiaRec's Generative AI Auto QA.
But the AI also looks at the conversation as a whole, to give contextual answers to more complex questions like the one mentioned earlier: "Did the agent actively listen to the customer's concerns and answer all the customer's questions without the customer needing to repeat themselves?" This answer requires the generative AI to properly analyze the whole conversation, as there could be multiple points within a call for this to happen. Unlike MiaRec's AI Auto QA, a rule-based QA would not be able to contextually analyze the call because it would only look at fragments of the call based on a set of rules.
Image: Screenshot of MiaRec's AI Assistant, offering contextual information for each question on a scorecard's results.
A beneficial feature when going through the scorecard is that the generative AI shows you from the transcript how it scored each answer. Simply click on the drop-down arrow to see the parts of the conversation that it pulled for its answer.
QA Dashboard
Image: Screenshot of a portion of MiaRec's QA Dashboard.
Since MiaRec's Generative AI Auto QA is analyzing 100% of calls, you can easily pick out your top and bottom performers. This allows you to choose which agents' calls can be used as good call examples, or have those agents conduct new-hire trainings. Also, you can quickly spot which agents need more training and in which areas, e.g., not following the call script.
The dashboard lets you filter by date range, department, etc., and compare to the previous period so you can easily follow the progress of agents to see if their training was effective, or if their performance starts to decline.
Instead of catching it at a quarterly review, or in one call out of a small percentage of randomly picked calls, poor performance can be caught quickly and remediated efficiently.
The dashboard allows you to even further filter calls by duration, inbound/outbound, agent, etc. You can even go further into a scorecard to see where in the call agents are lacking. Maybe the agents aren't using the proper greeting, or aren't properly verifying the accounts. Clicking into each scorecard gives you a more granular understanding of the results.
Image: Screenshot of a more granular view of MiaRec's QA Dashboard, showing average scores broken down by section of the scorecard and by agent.
Conclusion
With generative AI-driven auto QA, contact centers can now ensure consistent quality across all calls. This technology not only saves time but also provides a level of insight previously unattainable with manual evaluations. As contact centers continue to evolve, tools like these will be indispensable in maintaining high standards of customer service.
Generative AI-driven auto QA is a game-changer for contact centers. It offers a solution to the challenges of manual call evaluations, providing comprehensive, accurate, and contextual insights into agent performance. As technology continues to advance, contact centers that harness the power of innovations like these will be better equipped to meet the demands of the modern customer.
Share this
You May Also Like
These Related Stories
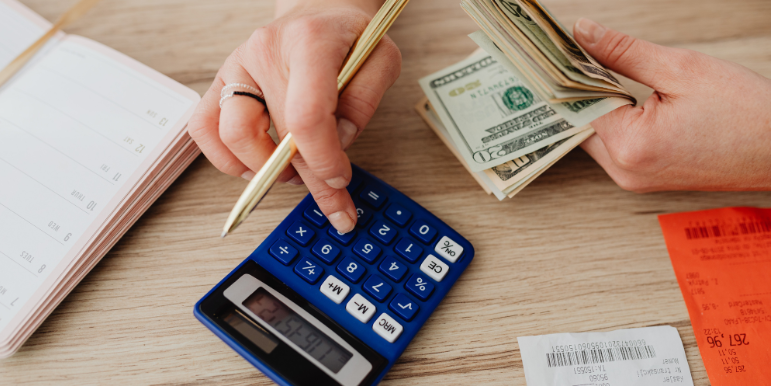
The ROI On Automated Quality Management For Contact Centers [Free Calculator]
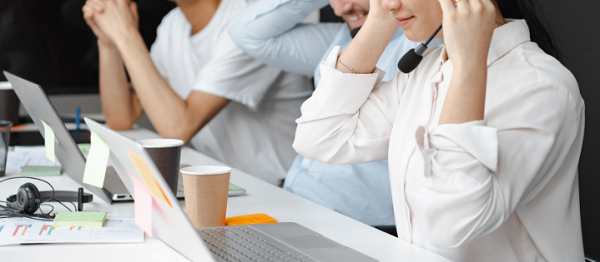
Maximize the ROI of Contact Center Voice Analytics: Top 5 Use Cases
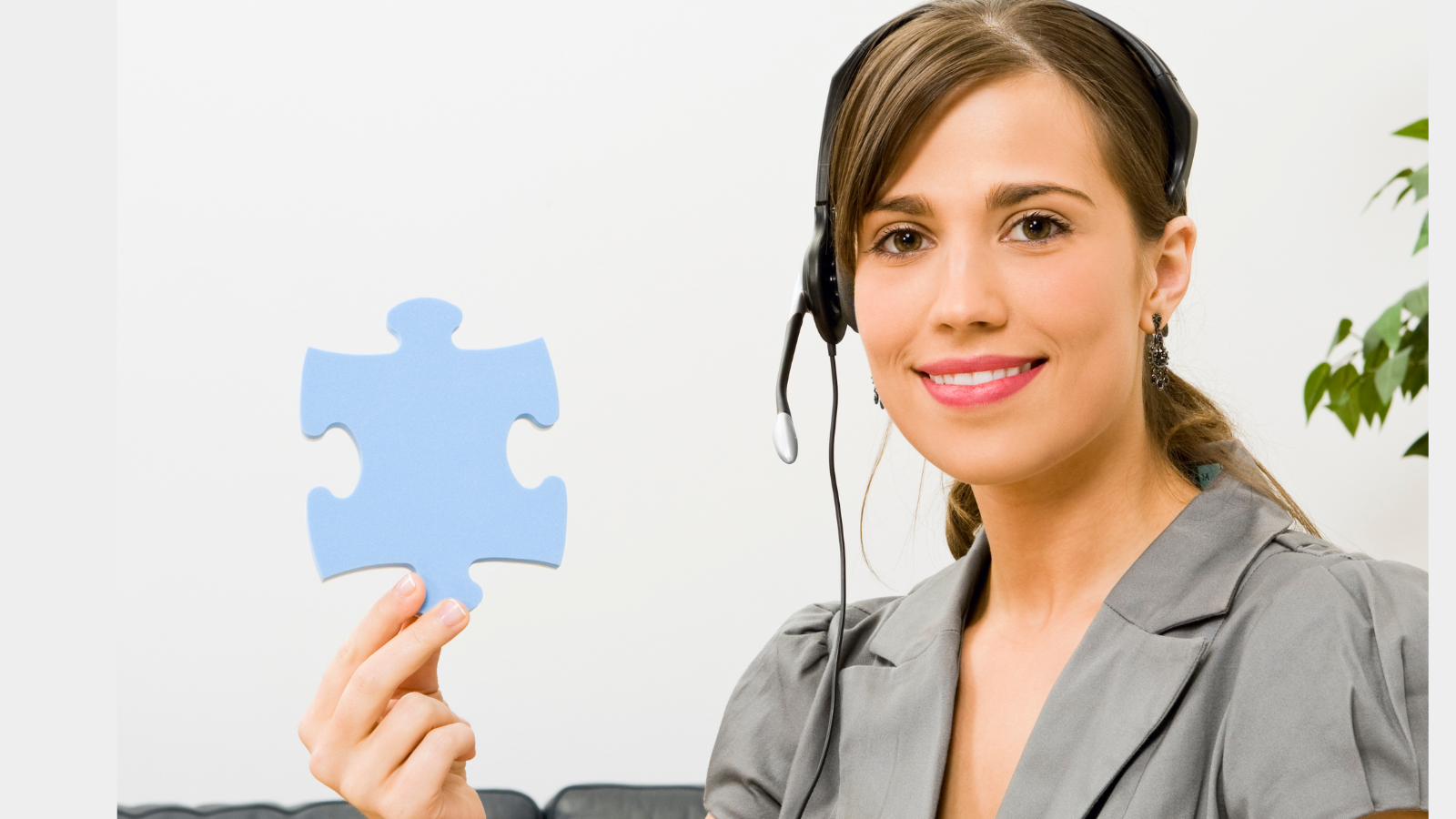