Results of a survey of data professionals show that about 1 out of 5 are women. Women are paid less than their male counterparts yet both women and men have similar levels of education. Ways of improving gender diversity in the field of data science are offered.
Even though women make up about half of the total workforce in the US, those numbers hide the disparities in some occupational domains. As you can see in Figure 1, while women make up about half of the life, physical and social science occupations in the US, they only account for 25% and 17% of the professionals in computer and mathematical occupations and architecture and engineering occupations, respectively.
Look at diversity numbers for some tech giants, and you will see that women make up a small part of the technology industry. In 2020, women made up 30% of the employees at Microsoft, 32% at Google, 45% at Amazon and 37% at Facebook. In fact, these numbers have only slightly improved, if at all, over the past 6 years.
How does gender diversity look in the data science world? Using data from LinkedIn, the World Economic Forum’s 2020 Global Gender Gap Report shows women make up only 26% of professionals in Data and AI.
I analyzed data from the 2020 Kaggle Machine Learning and Data Science survey in which they surveyed over 20,000 data professionals. That survey showed that, overall, 20% of the respondents were women (see Figure 2), a result somewhat comparable with the percent of women in high-tech companies in general. Gender disparity varied over job titles of data professionals. Women represented 24% (a high, mind you) of the data professionals who identified as a Statistician or Data Analyst. Women, on the other hand, represented only around 13% of data professionals who identified as a Product/Project Manager, Machine Learning Engineer, Software Engineer or Data(base) Engineer.
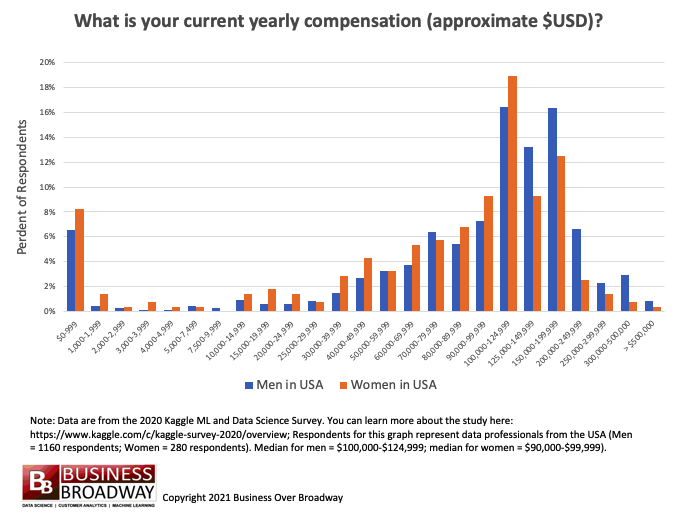
Salary Differences
Using data from 2018 and 2019, the US Census Bureau estimates that, for every dollar that men earn, women earn 81.6 cents.
We looked at differences between men and women data professionals in the US with respect to their salary. In line with the Census figures, women’s median salary in the US was $90,000-$99,999 while the men’s median salary was $100,000-$124,999 (see Figure 3). That comes out to women data professionals earning roughly 84 cents to every dollar that men earn.
Educational Background
Women and men in the field of data science do not differ with respect to their formal educational background (see Figure 3). For both genders, a majority of each held advanced degrees (i.e., Master’s: ~41%; Doctoral: ~12%) and around 35% held a Bachelor’s degree. Compared to the results of a similar analysis in 2015, the percent of data professionals holding Doctoral and Master’s degrees has declined while the percent of those holding Bachelor’s degrees has increased. The annual Stanford 2021 AI Index reports that women account for less than 19% of all AI and computer science PhD graduates in North America over the past 10 years.
In 2015, around 50% of data pros held a Master’s degree, 20% held a Doctoral degree and a quarter of them held a Bachelor’s degree. This shift in the educational makeup of those who are in the data analytics profession could be the result of more people moving into the field of data analytics at a young age. Also, the growth of universities that offer undergraduate degrees in the field of data science has exploded since 2015.
Not only do men and women data professionals possess similar educational degrees, they also possess the same skill set. In a prior study, I found that men and women possessed comparable proficiency in subject matter expertise, technology and programming and math/statistics.
Benefits of Gender Diversity
Gender diversity is good for business. In 2014, Gallup found that more gender diverse business units outperform their less gender diverse counterparts with respect to revenue and net profits. Also, in 2017, McKinsey reports that companies in the top quartile for gender diversity are 21% more likely to outperform companies in the bottom quartile.
It has been argued that improving gender diversity would help ameliorate the bias found in our AI algorithms. Because algorithms reflect the values of data professionals who create them, a diverse workforce would act as a gatekeeper to potential bias.
More simply put, if an organization’s leadership and workforce do not reflect the diverse range of customers it serves, its outputs will eventually be found to be substandard. Because learning algorithms are a part of larger systems composed of other technologies and the people who create them and implement them, bias can creep in anywhere in the pipeline. If the diversity within an organization’s pipeline is low at any point, the organization opens itself up to biases — including ones that are deep enough and, potentially, public enough that they could divide customers and eventually lead to obsolescence and failure. Some customers would stay, but others would leave.
~ Ayanna Howard and Charles Isbell from Diversity in AI: The Invisible Men and Women
What You Can Do to Improve Representation of Women in Data Science
Researchers Francine Berman and Philip Bourne proposed 10 ways you can improve gender diversity in data science. These changes come about through changing of the work culture and advocating for women’s representation in data science. You can see their specific tips in Figure 4.
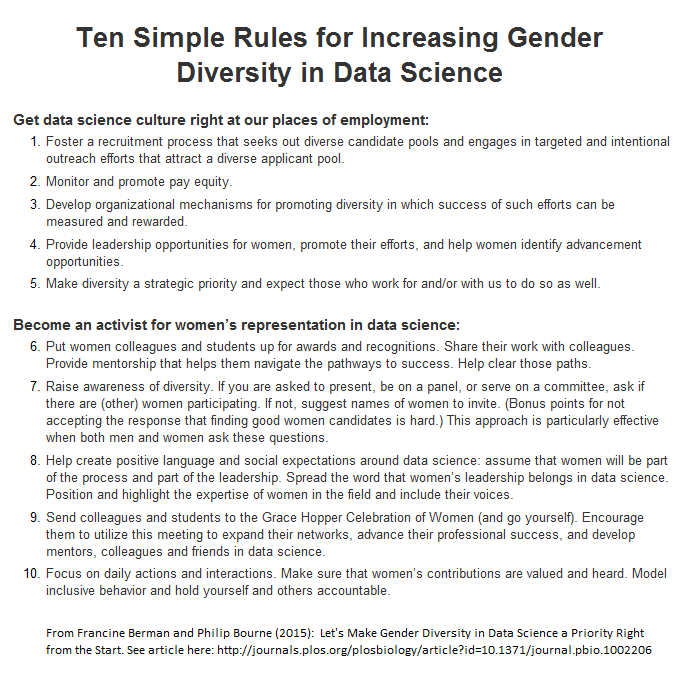
Summary
The data science, machine learning and AI professions have a long way to go to reach gender equality. The current analysis revealed low rates of women employees in all data-related roles. Gender inequality also revealed itself in salary differences of men and women, with women data professionals making Despite this gender disparity, we did not see any large gender differences with respect to educational background; both women and men achieved similar levels of education. Data professionals can take practical steps to improve the representation of women in data science, including changing the work culture around gender diversity and being an activist for women’s representation in data science.
Comments are closed.