Results of a survey of data professionals show that different data roles engage in different activities while at work. Data Scientists indicated that three activities make up an important part of their work, the most across all data roles. The top activities across all data roles were related to analyzing data to influence decisions and building prototypes.
The practice of data science is about extracting value from data to help inform decision making and improve algorithms. As such, data science requires three broad skill sets, including subject matter expertise, statistics/math and technology/programming. Because different data professionals possess diverse and complementary skill sets, the practice of data science requires a collaborative effort across different data professionals. But what exactly do different data professionals do at work? What broad activities make up their respective jobs?
Kaggle conducted a worldwide survey of over 20000 data professionals to learn about the state of data science and machine learning. The survey included the question, “Select any activities that make up an important part of your role at work: (Select all that apply).” They were given a list of six general activities from which to select.
Results showed that, on average, data professionals selected one activity. The percent of respondents that selected each activities is (see Figure 1):
- Analyze and understand data to influence product or business decisions (52%)
- Build prototypes to explore applying machine learning to new areas (32%)
- Build and/or run the data infrastructure that my business uses for storing, analyzing, and operationalizing data (27%)
- Experimentation and iteration to improve existing ML models (25%)
- Build and/or run a machine learning service that operationally improves my product or workflows (22%)
- Do research that advances the state of the art of machine learning (19%)
- None of these activities are an important part of my role at work (14%)
- Other (4%)
Differences across Job Titles
The number of activities that make up an important part of data professionals’ roles at work varied over job titles. Some job roles include a broader set of work activities compared to other job roles that included a more narrow set of activities. Specifically, most data professionals reported that only one activity that was an important part of their work; they included Business Analyst, DBA/Database Engineer, Data Analyst, Product/Project Manager, Software Engineer and Statistician.
Data professionals who reported two activities included Machine Learning Engineer, Data Engineer and Research Scientist. Data Scientists reported the highest number of work activities with three.
We selected a few job titles to illustrate the different activity profiles of data professionals (see Figure 2). Generally, we see that some job roles are broad with respect to their work activities (e.g., Data Scientist, Machine Learning Engineer) while others are narrow (e.g., Product/Project Manager, Business Analyst, DBA/Database Engineer).
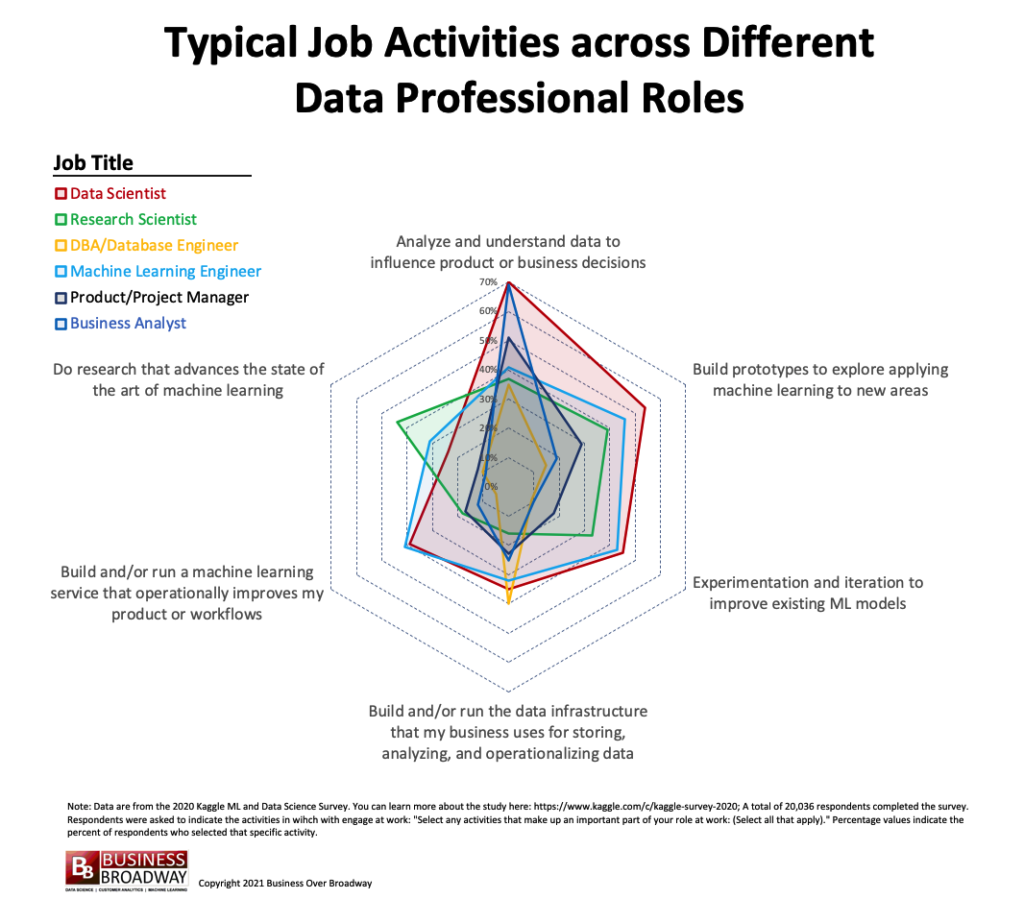
Figure 2. Percent of data professionals say activity is an important part of their role at work. Click image to enlarge.
While the top work activity was “Analyze and understand data” for most of the job titles (i.e., Business Analyst, Data Analyst, Data Engineer, Data Scientist, Product/Project Manager, Software Engineer and Statistician), the top activities for other job titles were:
- Research Scientist: Do research that advances the state of the art of ML
- DBA/Database Engineer: Build and/or run the data infrastructure
- Machine Learning Engineer: Build prototypes to explore applying ML
Summary
Data professionals are involved in different work activities. While many data pros are focused primarily on analyzing and understanding data (the top activity among data professionals), a few of them see their primary work around data infrastructure, building prototypes or conducting research to advance knowledge.
Respondents who self-identified as Data Scientists, on average, indicated that they are involved in the most number of activities at work (3), followed by Machine Learning Engineers, Data Engineers and Research Scientists who reported being involved in 2. The remaining data professionals are generally involved in one activity (Business Analyst, DBA/Database Engineer, Data Analyst, Product/Project Manager, Software Engineer and Statistician.
Not all data professionals are created equal. Results showed that the work activity profiles varied greatly across different data roles. While many of the respondents indicated that analysis and understanding of data to influence products/decisions was the top activity for them, a top activity for Research Scientists was doing research that advances the state of the art of machine learning. Additionally, the top activity for DBA/Database Engineers was building and/or running the data infrastructure. The activity profiles for each data professional (see Figure 2) gives us a sense that some jobs involve several important activities while others involve fewer (a single?) important activities.
The top work activity for data professional roles appears to be very practical and necessary to run day-to-day business operations. These top work activities included influencing business decisions, building prototypes to expand machine learning to new areas and improving ML models. The bottom activity was more about long-term understanding of machine learning reflected in conducting research to advance the state of the art of machine learning.
Different data roles possess different activity profiles. Top work activities tend to be associated with the skill sets of different data roles. Building/Running data infrastructure was the top activity for Data Engineers; doing research to advance the field of machine learning was a top activity for Research Scientists. These results are not surprising as we know that different data professionals have different skill sets. In prior research, I found that data professionals who self-identified as Researchers have a strong math/statistics/research skill set. Developers, on the other hand, have strong programming/technology skills. And data professionals who were Domain Experts have strong business-domain knowledge.
Remember that data professionals have their unique skill set that makes them a better fit for some data roles than others. When applying for data-related positions, it might be useful to look at the type of work activities for which you have experience (or are competent) and apply for the positions with corresponding job titles. For example, if you are proficient in running a data infrastructure, you might consider focusing on Data Engineer jobs. If you have a strong skill set related to research and statistics, you might be more likely to get a call back when applying for Research Scientist positions.
Data science and machine learning work really is a team sport. Getting data teams with members who have complementary skill sets who are capable of performing their specific job activities will likely improve the success rate of data science and machine learning projects.
Comments are closed.